With artificial intelligence’s ability to simulate human cognition and Data Science’s expertise in extracting actionable insights from vast datasets, we are headed towards a major transition that can redefine the boundaries on innovation.
Did you know that businesses leveraging AI and Data Science have reported up to a 40% increase in operational efficiency? This staggering statistic highlights the transformative potential of these technologies.
Today, we want all our readers to deeply understand how AI mimics human intelligence while Data Science harnesses data to drive decision-making.
The Technical Foundations: How AI and Data Science Differ in Approach
Recent advancements in AI and data science have been driven by breakthroughs in computational power, big data, and new algorithmic techniques. Here’s a more detailed look at their technical foundations:
1. Machine Learning (ML) and Data Science:
Machine learning is a subset of AI that enables machines to learn from data. However, its role in data science is distinct. While AI uses ML to build autonomous systems, data science uses ML primarily for prediction and classification.
A 2023 study from Stanford University highlighted those businesses leveraging machine learning for data science increased their predictive accuracy by 20%, leading to more efficient decision-making processes. This tells us how ML is used to predict outcomes rather than make autonomous decisions, a key differentiator between AI and data science.
2. Deep Learning (DL) in AI:
Deep learning, a subfield of machine learning, powers advanced AI applications like image recognition, self-driving cars, and language generation. In contrast, data science typically uses more traditional statistical methods. For example, deep learning models, like convolutional neural networks (CNNs), enable AI systems to achieve near-human levels of accuracy in tasks like image classification and object detection.
3. Data Pipelines: Real-Time vs. Batch Processing:
AI systems often require real-time data processing to adapt and improve in dynamic environments, such as in autonomous vehicles or AI-driven recommendation systems. On the other hand, data science workflows prioritize batch processing, where large datasets are cleaned, explored, and modeled in bulk to generate insights.
AI and Data Science: Foundational Definitions
AI is the brain behind machines that can think, learn, and adapt, transcending traditional programming to perform tasks that once seemed exclusively human. Imagine algorithms that can predict your needs before you even express them or systems that can diagnose diseases with pinpoint accuracy, this is the promise of AI.
Conversely, Data Science is the alchemy of the digital age, transforming raw data into gold. It combines statistical rigor with domain expertise to unearth insights that drive strategic decisions. In an era where data is generated at an unprecedented rate, the ability to sift through this information and extract meaningful patterns is invaluable.
What sets these fields apart is not just their functionality but their impact on society. AI automates and enhances human capabilities, while Data Science empowers us to make informed choices based on evidence rather than intuition. Together, they form a powerful duo that can solve complex problems, optimize operations, and even predict future trends.
Recent Research And Developments In AI And Data Science
Staying up-to-date with recent developments is key to understanding where AI and data science are headed. The latest research shows that both fields are moving towards more integrated, scalable, and ethically guided applications.
1. AI Scaling in Large Language Models (LLMs):
According to the 2024 AI Index Report by Stanford, large language models (LLMs) like GPT-4 and GPT-5 have set new benchmarks in natural language understanding, with applications spanning from content generation to code development. These models are not just limited to consumer applications but are making significant impacts in biomedical research, climate modeling, and financial forecasting.
LLMs are increasingly used in data science for automating data preprocessing tasks, such as generating summaries of large datasets and uncovering hidden patterns that might not be visible through traditional analysis methods.
2. Data Science and the Role of Big Data:
The rise of big data has been a pivotal force behind the growth of data science. A 2023 McKinsey report notes that organizations using advanced data science methods have seen a 40% improvement in operational efficiency. This includes the use of predictive analytics to optimize supply chains, enhance customer service, and manage risk.
How AI and Data Science Collaborate Across Industries
Both AI and data science are being applied in a variety of sectors, often complementing each other to deliver groundbreaking results. Here are industry-specific applications showcasing the synergy between AI and data science.
1. Healthcare: AI-Powered Diagnostics and Predictive Analytics
- AI in Diagnostics:
AI is revolutionizing diagnostics by analyzing medical images faster and more accurately than human experts. Deep learning algorithms, such as those employed by Google’s DeepMind, have been shown to detect early-stage cancers and eye diseases with over 95% accuracy.
- Data Science in Predictive Healthcare:
Data scientists leverage patient data to predict disease outbreaks, optimize treatment plans, and identify high-risk patients. Predictive models based on patient health records and clinical trial data are now integral to personalized medicine.
2. Finance: Risk Management and Fraud Detection
- AI In Finance:
AI-driven fraud detection systems can process vast amounts of transactional data in real-time, using machine learning models to flag suspicious activities. A recent case study from JP Morgan demonstrated that their AI system reduced fraud by 30% in the first year of implementation.
- Data Science in Financial Forecasting:
Data science plays a key role in developing predictive models that assess market trends, customer behavior, and portfolio performance. Using big data analytics, financial institutions are now able to make more informed investment decisions.
Careers: From Data Analyst To Chief Data Officer (CDO)
The journey from Data Analyst to Chief Data Officer (CDO) is a natural progression for those with strong technical, leadership, and strategic capabilities. Data analysts typically focus on analyzing data, building predictive models, and offering insights, but as they gain experience, many take on leadership roles that require managing data strategies across entire organizations.
As a CDO, the responsibilities expand to overseeing how data is collected, governed, analyzed, and used to drive business decisions. They develop the organization’s data policies, ensure data privacy and security compliance (e.g., GDPR), and manage teams that operationalize AI and Data Science initiatives.
This role increasingly focuses on aligning data strategy with business goals, leading to increased competitiveness and innovation.
According to a 2023 Gartner report, organizations with a CDO are 50% more likely to derive measurable business value from their data. The evolution from Data Analyst to CDO requires developing soft skills like strategic thinking, data governance knowledge, and team leadership.
Building expertise in data ethics, regulation compliance, and cross-departmental collaboration is critical for success at the CDO level, as is learning how to leverage AI and Data Science for scalable solutions that impact entire industries.
Bias, Transparency, And Privacy In AI And Data Science
As both AI and data science become more ingrained in daily life, ethical considerations are paramount. Understanding the risks and ensuring transparency in how algorithms and data are used is essential for building public trust.
1. Bias in AI Algorithms:
AI systems trained on biased datasets can perpetuate and amplify existing biases. This is particularly concerning in applications like hiring algorithms, lending decisions, or law enforcement. A 2023 MIT study found that facial recognition algorithms used in law enforcement were up to 30% less accurate in identifying individuals from minority groups.
Data scientists are tasked with identifying and mitigating bias in their models, a process known as Fair AI, ensuring that AI systems are fair, ethical, and unbiased.
2. Data Privacy and Security:
Both AI and data science rely on large datasets, often containing sensitive personal information. Ensuring privacy and security is a major challenge. The General Data Protection Regulation (GDPR) in the EU has set strict rules on how personal data can be collected and used, impacting how data scientists and AI practitioners operate.
Moreover, companies are increasingly adopting techniques like differential privacy to anonymize datasets, ensuring that AI and data science can coexist with robust privacy protections.
3. Explainability and Transparency in AI:
AI systems, especially deep learning models, are often criticized for being “black boxes” due to their complexity. Efforts to make these systems more interpretable are underway, with researchers developing methods like SHAP (SHapley Additive exPlanations) to explain model decisions. This is especially important in industries like healthcare, where understanding how an AI reached a decision is crucial for clinical validation.
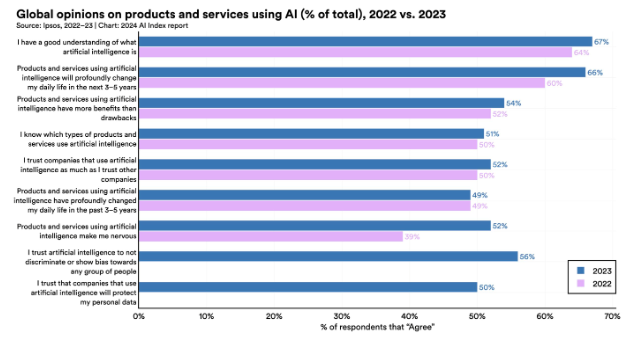
Parting Thoughts On Artificial Intelligence Vs Data Science
As we have explored, AI serves as a powerful tool to automate and enhance human capabilities, while Data Science acts as the analytical engine that transforms raw data into actionable insights.
The synergy between these two fields is evident across various industries, from AI in education to finance’s predictive analytics. However, with great power comes great responsibility. The ethical challenges surrounding bias, transparency, and data privacy must be addressed to ensure these technologies serve society equitably and responsibly.
As we look ahead, the integration of AI and Data Science will only deepen, driven by continuous advancements in technology and an ever-growing pool of data. Staying informed about these developments is crucial for leveraging their full potential.